- Добавил: literator
- Дата: 2-01-2024, 21:18
- Комментариев: 0
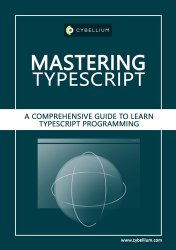
Автор: Cybellium Ltd
Издательство: Cybellium Ltd
Год: 2023
Страниц: 314
Язык: английский
Формат: epub
Размер: 10.2 MB
Shaping the Frontier of Web Development. Delve into the dynamic world of TypeScript—a powerful superset of jаvascript, designed to enhance productivity, facilitate large-scale applications, and push web development boundaries. Whether you're a frontend enthusiast, a backend guru, or someone in-between, "Mastering TypeScript" stands as your comprehensive companion in this journey. TypeScript is an open-source programming language that was developed and is maintained by Microsoft. The primary objective of TypeScript is to extend the capabilities of jаvascript by adding static types. The significance of this can be best understood when we compare it to jаvascript, a dynamically typed language. jаvascript, since its inception, has been one of the most popular programming languages for web development. However, as applications grow larger and more complex, certain limitations inherent to jаvascript become apparent. jаvascript being a dynamically typed language means that variable types are checked at runtime, and this lack of type safety can lead to runtime errors that are hard to track down and debug. TypeScript addresses this challenge by introducing static types. Static types allow developers to annotate their code with type information, which is then used by the TypeScript compiler for type checking at compile-time rather than runtime. This early error detection helps developers catch and fix errors more efficiently, resulting in more robust, reliable code.