- Добавил: literator
- Дата: 23-09-2023, 02:06
- Комментариев: 0
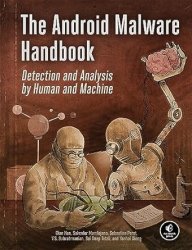
Автор: Qian Han, Salvador Mandujano, Sebastian Porst, V.S. Subrahmanian
Издательство: No Starch Press
Год: 2024
Страниц: 446
Язык: английский
Формат: epub
Размер: 25.6 MB
This groundbreaking guide to Android malware distills years of research by Machine Learning experts in academia and members of Meta and Google’s Android Security teams into a comprehensive introduction to detecting common threats facing the Android eco-system today. Explore the history of Android malware in the wild since the operating system first launched and then practice static and dynamic approaches to analyzing real malware specimens. Next, examine Machine Learning techniques that can be used to detect malicious apps, the types of classification models that defenders can implement to achieve these detections, and the various malware features that can be used as input to these models. Adapt these machine learning strategies to the identification of malware categories like banking trojans, ransomware, and SMS fraud. Running on over three billion devices worldwide, Android operates a significant portion of the Internet of Things and is the most popular operating system in history. This reach also makes it one of the most attractive targets for cybercriminals; indeed, as I write this, Android and its devices, stores, apps, and users face constant attacks from a wide range of actors, including well-resourced criminal and state-sponsored organizations.