- Добавил: literator
- Дата: 29-12-2023, 21:22
- Комментариев: 0
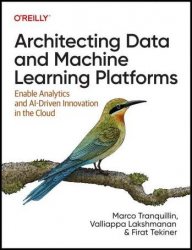
Автор: Marco Tranquillin, Valliappa Lakshmanan, Firat Tekiner
Издательство: O’Reilly Media, Inc.
Год: 2024
Страниц: 362
Язык: английский
Формат: pdf (true), epub (true)
Размер: 16.4 MB
What is a data platform? Why do you need it? What does building a data and Machine Learning (ML) platform involve? Why should you build your data platform on the cloud? This book starts by answering these common questions that arise when dealing with data and ML projects. We then lay out the strategic journey that we recommend you take to build data and ML capabilities in your business, show you how to execute on each step of that strategy, and wrap up all the concepts in a model data modernization case. All cloud architects need to know how to build data platforms that enable businesses to make data-driven decisions and deliver enterprise-wide intelligence in a fast and efficient way. This handbook shows you how to design, build, and modernize cloud native data and machine learning platforms using AWS, Azure, Google Cloud, and multicloud tools like Snowflake and Databricks.