- Добавил: kotmatros255
- Дата: 14-05-2022, 09:00
- Комментариев: 0
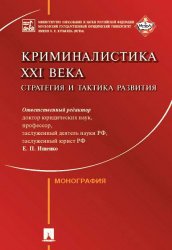
Автор: Отв. ред. Е.П. Ищенко
Издательство: Проспект
Год: 2016
ISBN: 978-5-392-20664-3
Формат: pdf
Страниц: 207
Размер: 12,8 Мб
Язык: русский
В монографии освещаются актуальные проблемы российской криминалистики как с позиций теории, так и с учетом богатого опыта правоприменительной деятельности, работы в оперативных, следственных либо экспертных подразделениях правоохранительных органов, который имеет каждый из соавторов.