- Добавил: SCART56
- Дата: 16-07-2023, 14:36
- Комментариев: 0
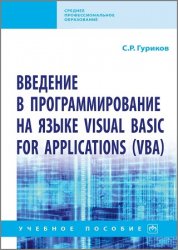
Название: Введение в программирование на языке Visual Basic for Applications (VBA)
Автор(ы): Гуриков С.Р.
Издательство: Инфра-М
Год: 2021
Страниц: 318
Формат: PDF
Размер: 67 Мб
Язык: русский
В учебном пособии рассмотрены основы алгоритмизации и программирования на языке Visual Basic for Applications (VBA), описано действие линейных, разветвляющихся и циклических структур. Большое внимание уделено программированию на основе функций и процедур, обработке одномерных и двумерных массивов, работе с файлами.