- Добавил: umkaS
- Дата: 17-04-2023, 10:38
- Комментариев: 0
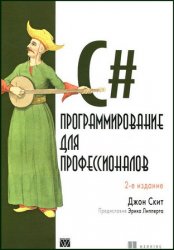
Автор: Скит Д.
Издательство: М.: Вильямc
Год: 2011 - 2-е издание
Cтраниц: 545
Формат: pdf
Размер: 20 мб
Язык: русский
Эта книга о языке C# версии 2 и выше. Язык C# 1, библиотеки инфраструктуры .NET Framework и общеязыковая исполняющая среда (CLR) рассматриваются только в связи с языком C#. Такое решение существенно отличает данную книгу от большинства книг по C# и .NET. Поскольку читатели имеют определенный объем знаний по языку C# 1, здесь не рассматривается материал, который, я полагаю, известен большинству.