- Добавил: SCART56
- Дата: 24-07-2023, 00:41
- Комментариев: 0
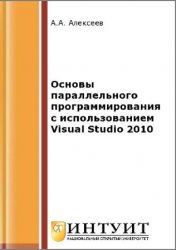
Название: Основы параллельного программирования с использованием Visual Studio 2010
Автор: Алексеев А.А.
Издательство: НОУ «Интуит»
Год: 2016
Страниц: 333
Формат: pdf
Размер: 25 mb
Данный курс, посвящен описанию создания многопоточных приложений в среде Visual Studio 2010 с использованием .NET Framework. В частности, какие библиотеки, и какие классы необходимы для создания многопоточных приложений, какие инструменты Visual Studio 2010 используются для отладки этих приложений.
На сегодняшний день, многоядерные процессоры используются не только в серверных платформах, но и в персональных компьютерах, которые позволяют нескольким потокам выполняться одновременно. И в будущем, число ядер в процессоре будет только увеличиваться. Уже сегодня можно использовать возможности аппаратного обеспечения – и распараллеливать код, чтобы распределить работу между несколькими процессорами. Ранее распараллеливание требовала низкого уровня обработки потоков и блокировок. Visual Studio 2010 и .NET Framework 4 поддерживают параллельное программирование путем предоставления новой среды CLR, новые типы библиотек классов и новые средства диагностики. Данные возможности упрощают разработку параллельных программ, так что можно написать эффективное и масштабируемое приложение без необходимости работать непосредственно с потоками или пулом потоков.