- Добавил: literator
- Дата: 20-12-2023, 18:33
- Комментариев: 0
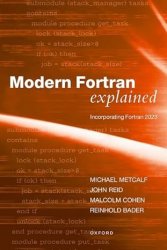
Автор: Michael Metcalf, John Reid, Malcolm Cohen
Издательство: Oxford University Press
Год: 2024
Страниц: 577
Язык: английский
Формат: pdf (true)
Размер: 10.2 MB
Fortran remains one of the principal programming languages used in high-performance scientific, numerical, and engineering computing. A series of significant revisions to the standard versions of the language have progressively enhanced its capabilities, and the current standard - Fortran 2023 - brings with it further additions and improvements. The language as defined by its most recent standards, with their introduction of object-oriented programming and of coarrays, is often referred to generically as 'Modern Fortran', and this term is increasingly used in the literature. Thus, we see that Fortran's particular advantages as a high-end numerical language, especially where arrays are the main form of data object and/or where complex arithmetic is involved, are still to the fore. It is able to attain the highest achievable optimization, mainly because multi-dimensional arrays are 'natural' objects and because its pointers are highly constrained. There is every sign that Modern Fortran will continue to be used to tackle major scientific computing problems in the next decade and beyond and will long remain a living tribute to its early pioneers. This new edition, written by experts in the field, three of whom have actively contributed to Fortran 2023, is thus a complete and authoritative description of Fortran in its latest form, with the intention that it remain the main reference work in the field.