- Добавил: literator
- Дата: 5-12-2023, 21:05
- Комментариев: 0
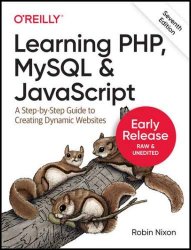
Автор: Robin Nixon
Издательство: O’Reilly Media, Inc.
Год: 2023-12-05
Страниц: 132
Язык: английский
Формат: pdf, epub, mobi
Размер: 10.8 MB
Build interactive, data-driven websites with the potent combination of open source technologies and web standards, even if you have only basic HTML knowledge. With the latest edition of this popular hands-on guide, you'll tackle dynamic web programming using the most recent versions of today's core technologies: PHP, MySQL, jаvascript, CSS, HTML5, jQuery, Node.js, and the powerful React library. Web designers will learn how to use these technologies together while picking up valuable web programming practices along the way, including how to optimize websites for mobile devices. You'll put everything together to build a fully functional social networking site suitable for both desktop and mobile browsers.