- Добавил: literator
- Дата: 27-03-2024, 01:23
- Комментариев: 0
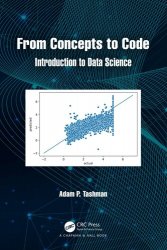
Автор: Adam P. Tashman
Издательство: CRC Press
Год: 2024
Страниц: 386
Язык: английский
Формат: pdf (true)
Размер: 17.4 MB
The breadth of problems that can be solved with Data Science is astonishing, and this book provides the required tools and skills fot a broad audience. The reader takes a journey into the forms, uses, and abuses of data and models, and learns how to critically examine each step. Python coding and data analysis skills are built from the ground up, with no prior coding experience assumed. The necessary background in Computer Science, mathematics, and statistics is provided in an approachable manner. This text uses Python because it is one of the most popular languages for Data Science, it supports the tasks we need to accomplish, and it is relatively easy to learn. It will teach Python by diving into problems with data and solving challenges. It will not methodically catalog what each operator does, what each control structure looks like, and so forth. There are excellent references for learning in this way, with suggestions given later. Finally, the book includes many exercises across different topics, with the hope that they are interesting and motivating. Each step of the Machine Learning lifecycle is discussed, from business objective planning to monitoring a model in production. This end-to-end approach supplies the broad view necessary to sidestep many of the pitfalls that can sink a Data Science project.