- Добавил: Natali26
- Дата: 13-01-2025, 22:26
- Комментариев: 0
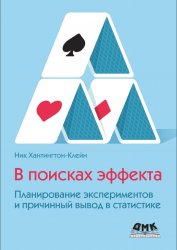
Автор: Хантингтон-Клейн Н.
Издательство: ДМК Пресс
Год: 2024
Формат: pdf
Страниц: 728
Размер: 12,70 Мб
Язык: русский
Эта книга посвящена стратегии поиска ответов на вопросы, в частности в области общественных наук, где данные наблюдений применяются для выявления причинного эффекта. Она состоит из двух частей, каждая из которых описывает разные подходы к задаче идентификации эффекта. Первая часть посвящена концепциям причинности с точки зрения наблюдаемого эффекта. Во второй части большое внимание уделяется процессам генерации данных и причинно-следственным диаграммам. Обсуждение идей подкреплено детальными иллюстрациями и пояснениями о том, что мы делаем с данными. Подробно раскрыта роль контрольных и инструментальных переменных в построении модели причинного вывода.