Validity, Reliability, and Significance: Empirical Methods for NLP and Data Science, 2nd Edition
- Добавил: literator
- Дата: 10-06-2024, 12:52
- Комментариев: 0
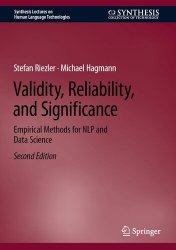
Автор: Stefan Riezler, Michael Hagmann
Издательство: Springer
Год: 2024
Страниц: 179
Язык: английский
Формат: pdf (true), epub
Размер: 25.7 MB
This book introduces empirical methods for Machine Learning with a special focus on applications in Natural Language Processing (NLP) and Data Science. The authors present problems of validity, reliability, and significance and provide common solutions based on statistical methodology to solve them. The book focuses on model-based empirical methods where data annotations and model predictions are treated as training data for interpretable probabilistic models from the well-understood families of generalized additive models (GAMs) and linear mixed effects models (LMEMs). Based on the interpretable parameters of the trained GAMs or LMEMs, the book presents model-based statistical tests such as a validity test that allows for the detection of circular features that circumvent learning. Furthermore, the book discusses a reliability coefficient using variance decomposition based on random effect parameters of LMEMs. Lastly, a significance test based on the likelihood ratios of nested LMEMs trained on the performance scores of two machine learning models is shown to naturally allow the inclusion of variations in meta-parameter settings into hypothesis testing, and further facilitates a refined system comparison conditional on properties of input data.
The book is self-contained with an appendix on the mathematical background of generalized additive models and linear mixed effects models as well as an accompanying webpage with the related R and Python code to replicate the presented experiments. The second edition also features a new hands-on chapter that illustrates how to use the included tools in practical applications.
Machine Learning is a research field that has been explored for several decades, and recently has begun to affect many areas of modern life under the reinvigorated label of Artificial Intelligence (AI). The goal of Machine Learning can be described as learning a mathematical function to make predictions on unseen test data, based on given training data, without explicit programmed instructions on how to perform the task. The methods employed for learning functional relationships between inputs and outputs heavily build on methods of mathematical optimization. While optimization problems are formalized as minimization of empirical risk functions on given training data, the important twist in Machine Learning is that it aims to optimize prediction performance in expectation, thus enabling generalization to unseen test data. The development and analysis of techniques for generalization is the topic of the dedicated sub-field of statistical learning theory. Statistical learning theory can be seen as the methodological basis of Machine Learning, and central concepts of statistical learning theory have been compared to Popper’s ideas of falsifiability of a scientific theory.
The focus of this book concerns empirical methods that allow for the assessment of the validity, reliability, and significance of prediction processes in NLP and data science. We cover prediction by data annotation, concerning the feature-label relation in the human data annotation process itself, and machine learning prediction, concerning predictions of labels by applications of machine learning models in NLP and Data Science. The book is organized in three main chapters on the topics of validity, reliability, and significance, respectively. Each chapter is organized by first discussing various theoretical and philosophical aspects of the respective concept. We take inspiration from these theoretical discussions to devise concrete tests that allow for the assessment of the validity, reliability, and significance.
Скачать Validity, Reliability, and Significance: Empirical Methods for NLP and Data Science, 2nd Edition

Внимание
Уважаемый посетитель, Вы зашли на сайт как незарегистрированный пользователь.
Мы рекомендуем Вам зарегистрироваться либо войти на сайт под своим именем.
Уважаемый посетитель, Вы зашли на сайт как незарегистрированный пользователь.
Мы рекомендуем Вам зарегистрироваться либо войти на сайт под своим именем.
Информация
Посетители, находящиеся в группе Гости, не могут оставлять комментарии к данной публикации.
Посетители, находящиеся в группе Гости, не могут оставлять комментарии к данной публикации.