Societal Impacts of Artificial Intelligence and Machine Learning
- Добавил: literator
- Дата: 24-03-2024, 19:31
- Комментариев: 0
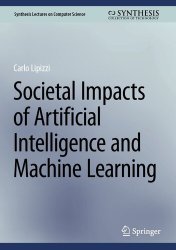
Автор: Carlo Lipizzi
Издательство: Springer
Серия: Synthesis Lectures on Computer Science
Год: 2024
Страниц: 160
Язык: английский
Формат: pdf (true), epub
Размер: 22.0 MB
This book goes beyond the current hype of expectations generated by the news on Artificial Intelligence and Machine Learning by analyzing realistic expectations for society, its limitations, and possible future scenarios for the use of this technology in our current society. Artificial Intelligence is one of the top topics today and is inflating expectations beyond what the technology can do in the foreseeable future. The future cannot be predicted, but the future of some elements of our society, such as technology, can be estimated. This book merges the modeling of human reasoning with the power of AI technology allowing readers to make more informed decisions about their personal or financial decisions or just being more educated on current technologies. This book presents a model that sketches potential future scenarios based on a discussion of the expectations today, the analysis of the current gap in the literature, and a view of possible futures in terms of technology and use cases. Specifically, this book merges literature on the technology aspects, the sociological impacts, and philosophical aspects.
Algorithms are the root science of AI. With minimal exceptions, all the new algorithms are around more “powerful” versions of what is called “artificial neural networks” (ANN). Artificial Neural Networks are a type of Machine Learning algorithm that is modeled after the structure and function of the human brain. They comprise interconnected nodes, called artificial neurons, that process information and make decisions. ANNs are used to analyze patterns and make predictions from large sets of data. ANNs are an interconnected web of decision-making units, mimicking how the brain comprises interconnected neurons. These interconnected units, or neurons, take inputs, process them, and then output the result. The neurons are organized in layers, with the input layer receiving the raw data, the hidden layers processing the data, and the output layer providing the result. The connections between the neurons, called edges, have a weight associated with them, which determines the strength of the connection. The weights are adjusted during the training process to optimize the network’s performance.
In current ML, the brain is represented by artificial neural networks (ANN). ANNs are a type of Machine Learning algorithm that is modeled after the structure and function of the human brain. They consist of layers of interconnected nodes, called artificial neurons, designed to simulate how neurons in the brain process and transmit information. Neural networks are used in various ML applications, such as image recognition, speech recognition, and natural language processing. In the vast majority of the existing applications, ANNs are mathematical models running on a traditional computer architecture (called “von Neuman architecture”). Neural networks are not the only way to represent brains. In a “symbolic” approach, brain could be represented by rules, taxonomies, or similar structures. In AI, algorithms and models that simulate cognitive processes such as perception, attention, memory, decision-making, and problem-solving often represent the mind. These algorithms and models are designed to replicate how the human mind works and are used to create intelligent systems such as expert systems, Natural Language Processing systems, and Machine Learning models.
Скачать Societal Impacts of Artificial Intelligence and Machine Learning
Внимание
Уважаемый посетитель, Вы зашли на сайт как незарегистрированный пользователь.
Мы рекомендуем Вам зарегистрироваться либо войти на сайт под своим именем.
Уважаемый посетитель, Вы зашли на сайт как незарегистрированный пользователь.
Мы рекомендуем Вам зарегистрироваться либо войти на сайт под своим именем.
Информация
Посетители, находящиеся в группе Гости, не могут оставлять комментарии к данной публикации.
Посетители, находящиеся в группе Гости, не могут оставлять комментарии к данной публикации.