Online Machine Learning: A Practical Guide with Examples in Python
- Добавил: literator
- Дата: 6-02-2024, 18:40
- Комментариев: 0
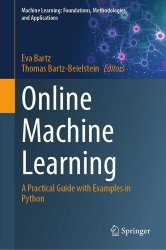
Автор: Eva Bartz, Thomas Bartz-Beielstein
Издательство: Springer
Серия: Machine Learning: Foundations, Methodologies, and Applications
Год: 2024
Страниц: 163
Язык: английский
Формат: pdf (true), epub
Размер: 19.0 MB
This book deals with the exciting, seminal topic of Online Machine Learning (OML). The content is divided into three parts: the first part looks in detail at the theoretical foundations of OML, comparing it to Batch Machine Learning (BML) and discussing what criteria should be developed for a meaningful comparison. The second part provides practical considerations, and the third part substantiates them with concrete practical applications.
The book is equally suitable as a reference manual for experts dealing with OML, as a textbook for beginners who want to deal with OML, and as a scientific publication for scientists dealing with OML since it reflects the latest state of research. But it can also serve as quasi OML consulting since decision-makers and practitioners can use the explanations to tailor OML to their needs and use it for their application and ask whether the benefits of OML might outweigh the costs.
OML will soon become practical; it is worthwhile to get involved with it now. This book already presents some tools that will facilitate the practice of OML in the future. A promising breakthrough is expected because practice shows that due to the large amounts of data that accumulate, the previous BML is no longer sufficient. OML is the solution to evaluate and process data streams in real-time and deliver results that are relevant for practice.
Chapter 1 describes the motivation for this book and the objective. It describes the drawbacks and limitations of BML and the need for OML. Chapter 2 gives an overview and evaluation of methods and algorithms with special focus on supervised learning (classification and regression). Chapter 3 describes procedures for drift detection. Updateability of OML procedures is discussed in Chap. 4. Chapter 5 explains procedures for the evaluation of OML methods. Chapter 6 deals with special requirements for OML. Possible OML applications are presented in Chap. 7 and evaluated by experts in official statistics. The availability of the algorithms in software packages, especially for R and Python, is presented in Chap. 8.
The computational effort required for updating the OML models, also in comparison to an algorithmically similar offline procedure (BML), is examined experimentally in Chap. 9. There, it is also discussed to what extent the model quality could be affected, especially in comparison to similar offline methods. Chapter 10 describes hyperparameter tuning for OML. Chapter 11 presents a summary and gives important recommendations for practitioners.
Скачать Online Machine Learning: A Practical Guide with Examples in Python

Внимание
Уважаемый посетитель, Вы зашли на сайт как незарегистрированный пользователь.
Мы рекомендуем Вам зарегистрироваться либо войти на сайт под своим именем.
Уважаемый посетитель, Вы зашли на сайт как незарегистрированный пользователь.
Мы рекомендуем Вам зарегистрироваться либо войти на сайт под своим именем.
Информация
Посетители, находящиеся в группе Гости, не могут оставлять комментарии к данной публикации.
Посетители, находящиеся в группе Гости, не могут оставлять комментарии к данной публикации.