Artificial Intelligence for Edge Computing
- Добавил: literator
- Дата: 23-12-2023, 07:36
- Комментариев: 0
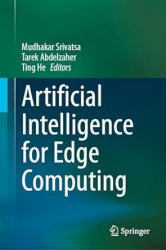
Автор: Mudhakar Srivatsa, Tarek Abdelzaher, Ting He
Издательство: Springer
Год: 2023
Страниц: 373
Язык: английский
Формат: pdf (true), epub
Размер: 41.7 MB
It is undeniable that the recent revival of Artificial Intelligence (AI) has significantly changed the landscape of science in many application domains, ranging from health to defense and from conversational interfaces to autonomous cars. With terms such as “Google Home”, “Alexa”, and “ChatGPT” becoming household names, the pervasive societal impact of AI is clear. Advances in AI promise a revolution in our interaction with the physical world, a domain where computational intelligence has always been envisioned as a transformative force toward a better tomorrow. Depending on the application family, this domain is often referred to as Ubiquitous Computing, Cyber-Physical Computing, or the Internet of Things. The underlying vision is driven by the proliferation of cheap embedded computing hardware that can be integrated easily into myriads of everyday devices from consumer electronics, such as personal wearables and smart household appliances, to city infrastructure and industrial process control systems. One common trait across these applications is that the data that the application operates on come directly (typically via sensors) from the physical world. Thus, from the perspective of communication network infrastructure, the data originate at the network edge. From a performance standpoint, there is an argument to be made that such data should be processed at the point of collection. Hence, a need arises for Edge AI -- a genre of AI where the inference, and sometimes even the training, are performed at the point of need, meaning at the edge where the data originate.
This book explores the challenges arising in Edge AI contexts. Some of these challenges (such as neural network model reduction to fit resource-constrained hardware) are unique to the edge environment. They need a novel category of solutions that do not parallel more typical concerns in mainstream AI. Others are adaptations of mainstream AI challenges to the edge space. An example is overcoming the cost of data labeling. The labeling problem is pervasive, but its solution in the IoT application context is different from other contexts. Importantly, before explaining further what the book is, let us add a few clarifications on what the book is not. This book is not a survey of the state of the art. With thousands of publications appearing in AI every year, such a survey is doomed to be incomplete on arrival. It is also not a comprehensive coverage of all the problems in the space of Edge AI. Different applications pose different challenges, and a more comprehensive coverage should be more application specific. Instead, this book covers some of the more endemic challenges across the range of IoT/CPS applications. To offer coverage in some depth, we opt to cover mainly one or a few representative solutions for each of these endemic challenges in sufficient detail, rather than broadly touching on all relevant prior work. The underlying philosophy is one of illustrating by example. The solutions are curated to offer insight into a way of thinking that characterizes Edge AI research and distinguishes its solutions from their more mainstream counterparts. The book is broken down into three parts: core problems, distributed problems, and other cross-cutting issues.
Contents:
Скачать Artificial Intelligence for Edge Computing

Внимание
Уважаемый посетитель, Вы зашли на сайт как незарегистрированный пользователь.
Мы рекомендуем Вам зарегистрироваться либо войти на сайт под своим именем.
Уважаемый посетитель, Вы зашли на сайт как незарегистрированный пользователь.
Мы рекомендуем Вам зарегистрироваться либо войти на сайт под своим именем.
Информация
Посетители, находящиеся в группе Гости, не могут оставлять комментарии к данной публикации.
Посетители, находящиеся в группе Гости, не могут оставлять комментарии к данной публикации.