Molecular Networking: Statistical Mechanics in the Age of AI and Machine Learning
- Добавил: literator
- Дата: 15-12-2023, 05:36
- Комментариев: 0
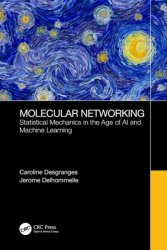
Автор: Caroline Desgranges, Jerome Delhommelle
Издательство: CRC Press
Год: 2024
Страниц: 249
Язык: английский
Формат: pdf (true)
Размер: 26.8 MB
The book builds on the analogy between social groups and assemblies of molecules to introduce the concepts of statistical mechanics, Machine Learning and Data Science. Applying a data analytics approach to molecular systems, we show how individual (molecular) features and interactions between molecules, or "communication" processes, allow for the prediction of properties and collective behavior of molecular systems - just as polling and social networking shed light on the behavior of social groups. Applications to systems at the cutting-edge of research for biological, environmental, and energy applications are also presented.
Social networks, Machine Learning, and Artificial Intelligence (AI) have become part of our daily lives. We live in an era where data analysis is present in all aspects of society and a driving force for many decisions that impact our present and future. While statistics have long played a significant role in numbers-driven domains, the development of novel machine learning algorithms, combined with the increase in computing performance and data storage, has led to a paradigm shift in how we approach and address challenges. For instance, in human health, the concept of precision medicine, which considers the individual features of a patient, has emerged as a promising alternative to one-size-fits-all medical treatments. Similarly, the sampling of opinion through polling methods had been for decades a staple of, for example, commercial and political analyses. It is now complemented by the analysis of data from social networks, which provide a window into human interactions and the interrelation between individual and collective responses.
This interrelation between individual features and collective properties also occurs at the microscopic level. Molecules interact with each other and respond to environmental stimuli, such as changes in external conditions or fields. As a result, they often exhibit a collective response and emergent properties. This is, for instance, the case when a group of molecules undergoes a phase transition, where the properties of the new phase dramatically depart from those of the initial phase. In other words, molecular networking can be thought of as equivalent to social networking for microscopic particles.
In writing this advanced textbook, we aim to provide a modern viewpoint on statistical mechanics. This field, which originated during the second half of the 19th century, is, by essence, data-driven. This is because statistical mechanics draws statistics on the distributions of microscopic quantities to predict macroscopic properties. In the book, we leverage the analogy between social and molecular networking to analyze the connection between individual properties and collective behavior. Part I of the book surveys the essential tools for this probabilistic analysis. This part successively draws concepts from mechanics (both classical and quantum) and statistics, characterizes the “communication processes” that take place at the microscopic scale, introduces the concept of statistical ensembles, discusses how the relation between the micro- and macroscopic worlds, and introduces how Machine Learning methods can provide further insight on molecular systems. Part II focuses on “polling” molecular systems at equilibrium to determine their properties. After discussing sampling approaches and the Monte Carlo simulation framework, Part II examines the impact of the environment on the nature (adiabatic vs. isothermal) of the ensemble before addressing how the onset of collective changes and emergence is captured by a central quantity, known as the partition function. Part II concludes with recent developments in statistical mechanics enabled by Machine Learning for interaction models, data interpolation and extrapolation, and the elucidation of transition pathways. Part III discusses molecular systems’ dynamics and time-dependent behavior, the determination of transport coefficients, and the systems’ response to external fields. It then opens on recent results in nonequilibrium statistical mechanics that shed light on the behavior of biological, active, and living systems before introducing how Machine Learning allows to classify transport behaviors, learn navigation strategies at the microscopic level, and identify new governing equations from data for far-from-equilibrium systems.
Reinforcement Learning (RL) is a framework that enables learning which sequence of actions is optimal to achieve success. This is done by passing on rewards, positive in case of success and negative otherwise, to the system. Rewards may be applied at the end of the trajectory, i.e., for the terminal state, or at various stages during the trajectory. What is necessary to realize, however, is that the feedback does not give any insight into what would have been the best trajectory or strategy, to achieve a goal. It is indeed up to the system to alter its trajectory or actions to achieve the goal. In other words, a single unfortunate step can ruin the entire trajectory, and it will be up to the system to identify the wrong step since the only feedback provided is a negative outcome. RL has recently been used to find efficient navigation strategies and show how particles and microorganisms learn to move optimally by trial and error.
Key features:
Draws on a data analytics approach of molecular systems.
Covers hot topics such as Artificial Intelligence and Machine Learning of molecular trends.
Contains applications to systems at the cutting-edge of research for biological, environmental and energy applications.
Discusses molecular simulation and links with other important, emerging techniques and trends in computational sciences and society.
Authors have a well-established track record and reputation in the field.
Скачать Molecular Networking: Statistical Mechanics in the Age of AI and Machine Learning

Внимание
Уважаемый посетитель, Вы зашли на сайт как незарегистрированный пользователь.
Мы рекомендуем Вам зарегистрироваться либо войти на сайт под своим именем.
Уважаемый посетитель, Вы зашли на сайт как незарегистрированный пользователь.
Мы рекомендуем Вам зарегистрироваться либо войти на сайт под своим именем.
Информация
Посетители, находящиеся в группе Гости, не могут оставлять комментарии к данной публикации.
Посетители, находящиеся в группе Гости, не могут оставлять комментарии к данной публикации.