Explainable Agency in Artificial Intelligence: Research and Practice
- Добавил: literator
- Дата: 13-12-2023, 17:01
- Комментариев: 0
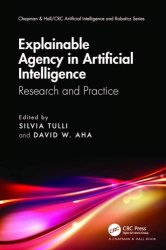
Автор: Silvia Tulli, David W. Aha
Издательство: CRC Press
Год: 2024
Страниц: 171
Язык: английский
Формат: pdf (true)
Размер: 10.1 MB
This book focuses on a subtopic of explainable AI (XAI) called explainable agency (EA), which involves producing records of decisions made during an agent’s reasoning, summarizing its behavior in human-accessible terms, and providing answers to questions about specific choices and the reasons for them. We distinguish explainable agency from interpretable machine learning (IML), another branch of XAI that focuses on providing insight (typically, for an ML expert) concerning a learned model and its decisions. In contrast, explainable agency typically involves a broader set of AI-enabled techniques, systems, and stakeholders (e.g., end users), where the explanations provided by EA agents are best evaluated in the context of human subject studies.
The chapters of this book explore the concept of endowing intelligent agents with explainable agency, which is crucial for agents to be trusted by humans in critical domains such as finance, self-driving vehicles, and military operations. This book presents the work of researchers from a variety of perspectives and describes challenges, recent research results, lessons learned from applications, and recommendations for future research directions in EA. The historical perspectives of explainable agency and the importance of interactivity in explainable systems are also discussed. Ultimately, this book aims to contribute to the successful partnership between humans and AI systems.
In contrast to the much broader topic of explainable AI (XAI), and its predominant focus on interpretable machine learning (IML), this book focuses on explainable agency. It addresses a gap in the literature on published volumes concerning this subtopic of XAI. This book presents the work of researchers focused on different facets of explainable agency, from diverse backgrounds, and describes challenges, new directions, recent research results, and lessons from applications.
Endowing agents with explainable agency is not only an academic exercise but a foremost priority in many real-world scenarios. In financial markets, self-driving vehicles, robot-assisted surgery, military operations, and other critical domains, whenever the behavior of these systems does not match human expectations (e.g., the car takes an unfamiliar turn), it is necessary to inform humans about how and why a certain decision has been taken. Providing these types of explanations impacts human trust towards the system and contributes to creating a shared mental model among the human and the AI system, leading to a more successful human-AI partnership.
Reinforcement learning (RL) is a computational approach to understanding and automating goal-directed learning and sequential decision making. It distinguishes itself from other machine learning fields through its emphasis on learning from direct sequential interactions of an agent in its environment, without the need for exemplary supervision or a complete model of the environment. The agent interacts with its environment over a series of time steps, where at each time step the agent receives an observation of the en- vironment and takes an action based on it. The agent accumulates rewards, positive or negative valued, from certain states it arrives at during its sequence. The agent’s goal is to maximize the cumulative reward it receives over time.
Features:
• Contributes to the topic of Explainable Artificial Intelligence (XAI)
• Focuses on the XAI subtopic of explainable agency
• Includes an introductory chapter, a survey, and five other original contributions
Скачать Explainable Agency in Artificial Intelligence: Research and Practice

Внимание
Уважаемый посетитель, Вы зашли на сайт как незарегистрированный пользователь.
Мы рекомендуем Вам зарегистрироваться либо войти на сайт под своим именем.
Уважаемый посетитель, Вы зашли на сайт как незарегистрированный пользователь.
Мы рекомендуем Вам зарегистрироваться либо войти на сайт под своим именем.
Информация
Посетители, находящиеся в группе Гости, не могут оставлять комментарии к данной публикации.
Посетители, находящиеся в группе Гости, не могут оставлять комментарии к данной публикации.