Building Recommendation Systems in Python and JAX: Hands-On Production Systems at Scale (Final)
- Добавил: literator
- Дата: 16-12-2023, 05:10
- Комментариев: 0
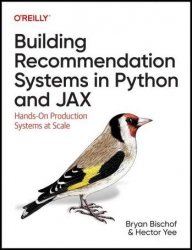
Автор: Bryan Bischof, Hector Yee
Издательство: O’Reilly Media, Inc.
Год: 2024
Страниц: 355
Язык: английский
Формат: pdf (true), epub (true)
Размер: 10.3 MB, 10.1 MB
Implementing and designing systems that make suggestions to users are among the most popular and essential machine learning applications available. Whether you want customers to find the most appealing items at your online store, videos to enrich and entertain them, or news they need to know, recommendation systems (RecSys) provide the way.
In this practical book, authors Bryan Bischof and Hector Yee illustrate the core concepts and examples to help you create a RecSys for any industry or scale. You'll learn the math, ideas, and implementation details you need to succeed. This book includes the RecSys platform components, relevant MLOps tools in your stack, plus code examples and helpful suggestions in PySpark, SparkSQL, FastAPI, and Weights & Biases.
Modern recommendation system (often abbreviated RecSys) designs are as diverse as the domains they serve. RecSys consist of the computer software architectures to implement and execute such product goals in addition to the algorithmic components of ranking. Methods for ranking recommendions can come from traditional statistical learning algorithms, linear algebraic inspirations, geometric considerations, and, of course, gradient based methods. Just as the algorithmic methods are diverse, so too are the modeling and evaluation considerations for recommending: personalized ranking, search recommendations, sequence modeling, and the scoring for all of the above are now need-to-know for the working ML Engineer in the space of recommendation systems.
If you’re an ML practitioner, you are probably aware of recommendation systems, and you may know one or two of the simplest modeling approaches and be able to speak intelligently about the relevant data structures and model architectures; however, RecSys frequently falls outside the core curriculum of data science and ML. Many senior data scientists with years of experience in the industry know little about actually building a recommendation system and may feel intimidated when the topic comes up. Despite drawing on similar foundations and skills as other ML problems, RecSys has a vibrant community with a fast-moving focus that can make it easy to relegate building recommendation systems to other data scientists who have already invested the time, or are willing to stay on top of the latest information.
The reason this book exists, is to break through those perceived barriers. Understanding recommendation systems at a practical level is not only useful for business cases requiring content to be served to users, but the underlying ideas of RecSys often bridge gaps between an incredibly diverse set of other types of ML. Take, for example, an article recommendation system that may utilize natural language processing (NLP) to find representations of the articles, sequential modeling to promote longer engagement, and contextual components to allow user queries to guide results. If you’re approaching the field from a purely academic interest, no matter what aspects of mathematics you’re interested in, sooner or later, there appears a link or application in RecSys!
You'll learn:
• The data essential for building a RecSys
• How to frame your data and business as a RecSys problem
• Ways to evaluate models appropriate for your system
• Methods to implement, train, test, and deploy the model you choose
• Metrics you need to track to ensure your system is working as planned
• How to improve your system as you learn more about your users, products, and business case
Скачать Building Recommendation Systems in Python and JAX: Hands-On Production Systems at Scale (Final)
True PDF:
True ePub:

Внимание
Уважаемый посетитель, Вы зашли на сайт как незарегистрированный пользователь.
Мы рекомендуем Вам зарегистрироваться либо войти на сайт под своим именем.
Уважаемый посетитель, Вы зашли на сайт как незарегистрированный пользователь.
Мы рекомендуем Вам зарегистрироваться либо войти на сайт под своим именем.
Информация
Посетители, находящиеся в группе Гости, не могут оставлять комментарии к данной публикации.
Посетители, находящиеся в группе Гости, не могут оставлять комментарии к данной публикации.