Methods and Applications of Autonomous Experimentation
- Добавил: literator
- Дата: 18-11-2023, 18:24
- Комментариев: 0
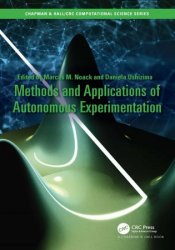
Автор: Marcus M. Noack, Daniela Ushizima
Издательство: CRC Press
Серия: Chapman & Hall/CRC Computational Science
Год: 2024
Страниц: 445
Язык: английский
Формат: pdf (true)
Размер: 41.3 MB
Autonomous Experimentation is poised to revolutionize scientific experiments at advanced experimental facilities. Whereas previously, human experimenters were burdened with the laborious task of overseeing each measurement, recent advances in mathematics, machine learning and algorithms have alleviated this burden by enabling automated and intelligent decision-making, minimizing the need for human interference. Illustrating theoretical foundations and incorporating practitioners’ first-hand experiences, this book is a practical guide to successful Autonomous Experimentation.
Despite the field’s growing potential, there exists numerous myths and misconceptions surrounding Autonomous Experimentation. Combining insights from theorists, Machine Learning engineers and applied scientists, this book aims to lay the foundation for future research and widespread adoption within the scientific community.
Just like so many other topics that have been adopted into the realm of Machine Learning and AI—Deep Learning, digital twins, active learning, and so on—Autonomous Experimentation has become a fuzzy, ill-defined ideal everyone wants, but seemingly no one can deliver. The main reason for that is the missing, inherent meaning of the term “Autonomous Experimentation”. In this book, we want to separate the practical methods and applications from the buzz and hype surrounding the term.
Autonomous Experimentation (AE) is an emerging paradigm for accelerating scientific discovery, leveraging Artificial Intelligence and Machine Learning methods to automate the entire experimental loop, including the decision-making step. AE combines advancements in hardware automation, data analytics, modeling, and active learning to augment a scientific instrument, enabling it to autonomously explore the search space corresponding to a problem of interest. AE can be deployed quite easily in any context where automation is feasible, by connecting a decision-making algorithm in between data analysis and machine-command modules. By its nature, AE is an application of Artificial Intelligence (AI) and Machine Learning (ML) methods to experimental science, since only AI/ML decision-making algorithms can provide the necessary sophistication to direct experimental execution intelligently—that is, in response to the iterative improvement in understanding one accumulates while researching a problem.
This book is particularly useful for members of the scientific community looking to improve their research methods but also contains additional insights for students and industry professionals interested in the future of the field.
Скачать Methods and Applications of Autonomous Experimentation

Внимание
Уважаемый посетитель, Вы зашли на сайт как незарегистрированный пользователь.
Мы рекомендуем Вам зарегистрироваться либо войти на сайт под своим именем.
Уважаемый посетитель, Вы зашли на сайт как незарегистрированный пользователь.
Мы рекомендуем Вам зарегистрироваться либо войти на сайт под своим именем.
Информация
Посетители, находящиеся в группе Гости, не могут оставлять комментарии к данной публикации.
Посетители, находящиеся в группе Гости, не могут оставлять комментарии к данной публикации.