Advanced Mathematical Applications in Data Science
- Добавил: literator
- Дата: 1-10-2023, 16:49
- Комментариев: 0
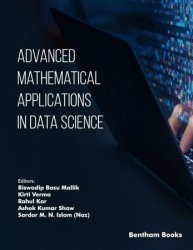
Автор: Biswadip Basu Mallik, Kirti Verma, Rahul Kar, Ashok Kumar Shaw
Издательство: Bentham Books
Год: 2023
Страниц: 223
Язык: английский
Формат: pdf (true), epub
Размер: 27.1 MB
Advanced Mathematical Applications in Data Science comprehensively explores the crucial role mathematics plays in the field of Data Science. Each chapter is contributed by scientists, researchers, and academicians. The 13 chapters cover a range of mathematical concepts utilized in Data Science, enabling readers to understand the intricate connection between mathematics and data analysis. The book covers diverse topics, including, Machine Learning models, the Kalman filter, data modeling, artificial neural networks, clustering techniques, and more, showcasing the application of advanced mathematical tools for effective data processing and analysis. With a strong emphasis on real-world applications, the book offers a deeper understanding of the foundational principles behind data analysis and its numerous interdisciplinary applications. This reference is an invaluable resource for graduate students, researchers, academicians, and learners pursuing a research career in mathematical computing or completing advanced data science courses.
An Artificial Neural Network (ANN) is a data processing paradigm inspired by the way organic nervous systems, such as the brain, process data. The innovative structure of the information processing system is a crucial component of this paradigm. It is made up of a huge number of highly linked processing components (neurons) that work together to solve issues. Neural networks handle data in the same manner that the human brain does. The network is made up of several densely linked processing units (neurons) that operate in parallel to solve a given problem. They are unable to be programmed to execute a specific activity. ANN, like humans, learns by example. Through a learning process, an ANN is trained for a specific application, such as pattern recognition or data categorization. In biological systems, learning includes changes to the synaptic connections that occur between neurons. This is also true for ANNs. Artificial Neural Networks are used for classification, regression, and grouping. Stages of image processing are classified as preprocessing, feature extraction, and classification. It can be utilized later in the process. ANN should be provided with features and output should be classified. This paper provides an overview of Artificial Neural Networks (ANN), their operation, and training. It also explains the application and its benefits. Artificial Neural Network has been used to classify the MNIST dataset.
The model is developed by employing Machine Learning techniques on Big Data platforms. Customer churn is one of the most critical issues, especially in high investment telecommunication companies. Accordingly, the companies are looking for ways to predict potential customers to churn and take necessary actions to reduce the churn. To accomplish the objective of the study, it first compares eight Machine Learning techniques, i.e., ridge classifier, gradient booster, adaptive boosting, bagging classifier, k-nearest neighbour (kNN), decision tree, logistic regression, and random forest. By using five evaluation performance metrics (i.e., accuracy, AUC score, precision score, recall score, and the F score), kNN is selected since it outperforms other techniques. Second, the selected technique is used to predict the likelihood of customers churning.
Key Features:
- Comprehensive coverage of advanced mathematical concepts and techniques in data science.
- Contributions from established scientists, researchers, and academicians.
- Real-world case studies and practical applications of mathematical methods.
- Focus on diverse areas, such as image classification, carbon emission assessment, customer churn prediction, and healthcare data analysis.
- In-depth exploration of data science's connection with mathematics, computer science, and artificial intelligence.
- Scholarly references for each chapter.
- Suitable for readers with high school-level mathematical knowledge, making it accessible to a broad audience in academia and industry.
Скачать Advanced Mathematical Applications in Data Science

Внимание
Уважаемый посетитель, Вы зашли на сайт как незарегистрированный пользователь.
Мы рекомендуем Вам зарегистрироваться либо войти на сайт под своим именем.
Уважаемый посетитель, Вы зашли на сайт как незарегистрированный пользователь.
Мы рекомендуем Вам зарегистрироваться либо войти на сайт под своим именем.
Информация
Посетители, находящиеся в группе Гости, не могут оставлять комментарии к данной публикации.
Посетители, находящиеся в группе Гости, не могут оставлять комментарии к данной публикации.