Representation Learning for Natural Language Processing, Second Edition
- Добавил: literator
- Дата: 24-08-2023, 08:08
- Комментариев: 0
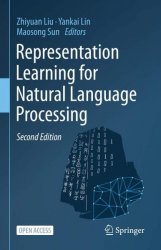
Автор: Zhiyuan Liu, Yankai Lin, Maosong Sun
Издательство: Springer
Год: 2023
Страниц: 535
Язык: английский
Формат: pdf (true)
Размер: 14.7 MB
This book provides an overview of the recent advances in representation learning theory, algorithms, and applications for natural language processing (NLP), ranging from word embeddings to pre-trained language models. It is divided into four parts. Part I presents the representation learning techniques for multiple language entries, including words, sentences and documents, as well as pre-training techniques.
Part II then introduces the related representation techniques to NLP, including graphs, cross-modal entries, and robustness. Part III then introduces the representation techniques for the knowledge that are closely related to NLP, including entity-based world knowledge, sememe-based linguistic knowledge, legal domain knowledge and biomedical domain knowledge. Lastly, Part IV discusses the remaining challenges and future research directions.
The theories and algorithms of representation learning presented can also benefit other related domains such as machine learning, social network analysis, semantic Web, information retrieval, data mining and computational biology. This book is intended for advanced undergraduate and graduate students, post-doctoral fellows, researchers, lecturers, and industrial engineers, as well as anyone interested in representation learning and natural language processing.
As compared to the first edition, the second edition (1) provides a more detailed introduction to representation learning in Chapter 1; (2) adds four new chapters to introduce pre-trained language models, robust representation learning, legal knowledge representation learning and biomedical knowledge representation learning; (3) updates recent advances in representation learning in all chapters; and (4) corrects some errors in the first edition. The new contents will be approximately 50%+ compared to the first edition.
Prerequisites:
This book is designed for advanced undergraduate and graduate students, post-doctoral fellows, researchers, lecturers, industrial engineers, and anyone interested in representation learning, NLP, knowledge engineering, and AI. This is not a textbook, and we don’t introduce basic knowledge. We expect the readers to have prior knowledge of Probability, Linear Algebra, and Machine Learning. We recommend that readers interested in NLP read the first part (Chaps. 1–5) in sequence. The remaining parts can be read according to readers’ interests. Many areas of representation learning are still evolving quickly. Hence, we list some books, reviews, and resources at the end of each chapter for readers to learn more about the topics.
Скачать Representation Learning for Natural Language Processing, Second Edition

Внимание
Уважаемый посетитель, Вы зашли на сайт как незарегистрированный пользователь.
Мы рекомендуем Вам зарегистрироваться либо войти на сайт под своим именем.
Уважаемый посетитель, Вы зашли на сайт как незарегистрированный пользователь.
Мы рекомендуем Вам зарегистрироваться либо войти на сайт под своим именем.
Информация
Посетители, находящиеся в группе Гости, не могут оставлять комментарии к данной публикации.
Посетители, находящиеся в группе Гости, не могут оставлять комментарии к данной публикации.