Machine Learning under Resource Constraints, Volume 2: Discovery in Physics
- Добавил: literator
- Дата: 12-02-2023, 21:08
- Комментариев: 0

Автор: Katharina Morik, Wolfgang Rhode
Издательство: De Gruyter
Год: 2023
Страниц: 364
Язык: английский
Формат: pdf (true), epub
Размер: 56.9 MB
Machine Learning under Resource Constraints addresses novel Machine Learning algorithms that are challenged by high-throughput data, by high dimensions, or by complex structures of the data in three volumes. Resource constraints are given by the relation between the demands for processing the data and the capacity of the computing machinery. The resources are runtime, memory, communication, and energy. Hence, modern computer architectures play a significant role. Novel Machine Learning algorithms are optimized with regard to minimal resource consumption. Moreover, learned predictions are executed on diverse architectures to save resources. It provides a comprehensive overview of the novel approaches to Machine Learning research that consider resource constraints, as well as the application of the described methods in various domains of science and engineering.
Volume 2 covers Machine Learning for knowledge discovery in particle and astroparticle physics. Their instruments, e.g., particle detectors or telescopes, gather petabytes of data. Here, Machine Learning is necessary not only to process the vast amounts of data and to detect the relevant examples efficiently, but also as part of the knowledge discovery process itself. The physical knowledge is encoded in simulations that are used to train the machine learning models. At the same time, the interpretation of the learned models serves to expand the physical knowledge. This results in a cycle of theory enhancement supported by Machine Learning.
Artificial Intelligence (AI) began as a model of cognitive processes. This applies to several levels: the neurons at the subsymbolic level, models of learning and decision-making at the individual level, and the process of scientific discovery at the epistemological level, which is our concern here. As a model of the scientific process, Machine Learning started early on to discover regularities in data, generate hypotheses, and test them. Statistics is employed for hypothesis testing, but Machine Learning has a much broader view of the overall process of modeling, including hypothesis generation, self-supervision of the learning algorithm, creating representations, and the generation of new data by conducting experiments automatically. Approaches to enhance given or learned models, called “explanation-based learning” were also discovered as part of the field.
Contents:
1 Introduction
2 Challenges in Particle and Astroparticle Physics
3 Key Concepts in Machine Learning and Data Analysis
4 Data Acquisition and Data Structure
5 Monte Carlo Simulations
6 Data Storage and Access
7 Monitoring and Feature Extraction
8 Event Property Estimation and Signal Background Separation
9 Deep Learning Applications
10 Inverse Problems
Bibliography
Index
Скачать Machine Learning under Resource Constraints, Volume 2: Discovery in Physics
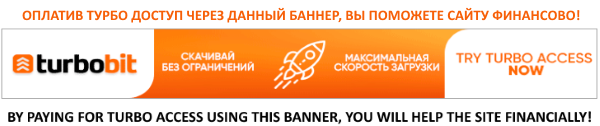
Внимание
Уважаемый посетитель, Вы зашли на сайт как незарегистрированный пользователь.
Мы рекомендуем Вам зарегистрироваться либо войти на сайт под своим именем.
Уважаемый посетитель, Вы зашли на сайт как незарегистрированный пользователь.
Мы рекомендуем Вам зарегистрироваться либо войти на сайт под своим именем.
Информация
Посетители, находящиеся в группе Гости, не могут оставлять комментарии к данной публикации.
Посетители, находящиеся в группе Гости, не могут оставлять комментарии к данной публикации.