Reinforcement Learning Aided Performance Optimization of Feedback Control Systems
- Добавил: literator
- Дата: 25-04-2021, 16:38
- Комментариев: 0
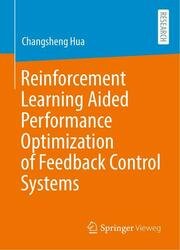
Автор: Changsheng Hua
Издательство: Springer
Год: 2021
Страниц: 139
Язык: английский
Формат: pdf (true)
Размер: 10.1 MB
Changsheng Hua proposes two approaches, an input/output recovery approach and a performance index-based approach for robustness and performance optimization of feedback control systems. For their data-driven implementation in deterministic and stochastic systems, the author develops Q-learning and natural actor-critic (NAC) methods, respectively. Their effectiveness has been demonstrated by an experimental study on a brushless direct current motor test rig.
Reinforcement learning (RL) is a branch of Machine Learning (ML) concerned with how agents take actions by interacting with their environment to maximize some notion of cumulative reward. It is a powerful tool to solve optimization problems in a data-driven manner. There have been a lot of remarkable results over the last few decades about applications of RL to performance optimization such as playing Atari games and Go with super-human performance, and training robotics to learn primitive skills. Meanwhile, there have been also a lot of studies on applications of RL to controller design in the control community. This includes learning of state feedback controllers and H ∞ controllers for linear systems, learning of optimal controllers for nonlinear systems. All of them suggest the potential of RL in the application of performance optimization of feedback control systems.
Why Reinforcement Learning Aided Performance Optimization of Feedback Control Systems? To deal with performance degradation, fault-tolerant control methods have been widely developed. Generally, they fall into two categories 1) model-based, 2) data-driven. One kind of model-based methods is to pre-design a set of controllers for a group of faulty scenarios, so that once any of them is detected, the corresponding controller is switched into operation to ensure stability and maintain acceptable performance. The other kind of model-based methods is to design adaptive controllers, such as model following or sliding mode controllers, whose parameters change in accord with plant changes, so that performance degradation is not “visible” from the plant input/output (I/O) responses. However, due to their innate model-based nature, these performance optimization methods cannot be easily transplanted among different systems.
Скачать Reinforcement Learning Aided Performance Optimization of Feedback Control Systems
Внимание
Уважаемый посетитель, Вы зашли на сайт как незарегистрированный пользователь.
Мы рекомендуем Вам зарегистрироваться либо войти на сайт под своим именем.
Уважаемый посетитель, Вы зашли на сайт как незарегистрированный пользователь.
Мы рекомендуем Вам зарегистрироваться либо войти на сайт под своим именем.
Информация
Посетители, находящиеся в группе Гости, не могут оставлять комментарии к данной публикации.
Посетители, находящиеся в группе Гости, не могут оставлять комментарии к данной публикации.