Image Fusion in Remote Sensing: Conventional and Deep Learning Approaches
- Добавил: literator
- Дата: 6-04-2021, 14:41
- Комментариев: 0
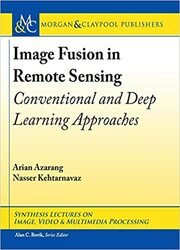
Автор: Arian Azarang, Nasser Kehtarnavaz
Издательство: Morgan & Claypool
Год: 2021
Страниц: 95
Язык: английский
Формат: pdf (true)
Размер: 43.8 MB
Image fusion in remote sensing or pansharpening involves fusing spatial (panchromatic) and spectral (multispectral) images that are captured by different sensors on satellites. This book addresses image fusion approaches for remote sensing applications. Both conventional and Deep Learning approaches are covered. First, the conventional approaches to image fusion in remote sensing are discussed. These approaches include component substitution, multi-resolution, and model-based algorithms. Then, the recently developed Deep Learning (DL) approaches involving single-objective and multi-objective loss functions are discussed. Experimental results are provided comparing conventional and Deep Learning approaches in terms of both low-resolution and full-resolution objective metrics that are commonly used in remote sensing. The book is concluded by stating anticipated future trends in pansharpening or image fusion in remote sensing.
In chapter 4, recently developed Deep Learning approaches for remote sensing image fusion are presented. First, different Deep Learning networks used are described. In addition, their training procedure is described. Then, the way Deep Learning models are applied to the fusion problem is discussed in detail. To this end, several representative methods are first mentioned.
Deep Learning is a subset of Machine Learning (ML) algorithms that use a set of layers of processing elements for nonlinear data processing in a supervised or an unsupervised manner. Front layers of a Deep Learning model extract low-level features of input image data while deeper layers extract high-level features. Backend layers of a Deep Learning model perform classification or regression similar to classical pattern recognition. In other words, both feature extraction and pattern recognition are done together in a Deep Learning model while in conventional Machine Learning, feature extraction and classification are done separately.
In general, three main deep learning architectures are considered:
- generative,
- discriminative, and
- hybrid.
Pre-trained layers are used by some of the layers of several Deep Learning solutions. This so-called transfer learning approach reduces the challenge of learning low-level hidden layers. Each layer may be pre-trained (DNNs) and later included in fine-tuning steps in the overall model. Discriminative deep neural networks achieve differential computing capacity by stacking the output of each layer with the original data or using a number of combinations. Such Deep Learning models consider the outputs as a conditional distribution over all possible label sequences for a given input sequence, which is then optimized based on an objective function. Hybrid Deep Learning models combine the properties of the generative and discriminative architectures.
Скачать Image Fusion in Remote Sensing: Conventional and Deep Learning Approaches
Внимание
Уважаемый посетитель, Вы зашли на сайт как незарегистрированный пользователь.
Мы рекомендуем Вам зарегистрироваться либо войти на сайт под своим именем.
Уважаемый посетитель, Вы зашли на сайт как незарегистрированный пользователь.
Мы рекомендуем Вам зарегистрироваться либо войти на сайт под своим именем.
Информация
Посетители, находящиеся в группе Гости, не могут оставлять комментарии к данной публикации.
Посетители, находящиеся в группе Гости, не могут оставлять комментарии к данной публикации.