Graph Representation Learning (Synthesis Lectures on Artificial Intelligence and Machine Learning)
- Добавил: literator
- Дата: 29-03-2021, 20:22
- Комментариев: 0
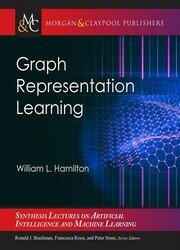
Автор: William L. Hamilton
Издательство: Morgan & Claypool
Серия: Synthesis Lectures on Artificial Intelligence and Machine Learning
Год: 2020
Страниц: 161
Язык: английский
Формат: pdf (true)
Размер: 10.1 MB
Graph-structured data is ubiquitous throughout the natural and social sciences, from telecommunication networks to quantum chemistry. Building relational inductive biases into Deep Learning (DL) architectures is crucial for creating systems that can learn, reason, and generalize from this kind of data. Recent years have seen a surge in research on graph representation learning, including techniques for deep graph embeddings, generalizations of convolutional neural networks to graph-structured data, and neural message-passing approaches inspired by belief propagation. These advances in graph representation learning have led to new state-of-the-art results in numerous domains, including chemical synthesis, 3D vision, recommender systems, question answering, and social network analysis.
Machine Learning (ML) is inherently a problem-driven discipline. We seek to build models that can learn from data in order to solve particular tasks, and machine learning models are often categorized according to the type of task they seek to solve: Is it a supervised task, where the goal is to predict a target output given an input datapoint? Is it an unsupervised task, where the goal is to infer patterns, such as clusters of points, in the data?
Machine Learning with graphs is no different, but the usual categories of supervised and unsupervised are not necessarily the most informative or useful when it comes to graphs. In this section we provide a brief overview of the most important and well-studied machine learning tasks on graph data. As we will see, "supervised" problems are popular with graph data, but Machine Learning problems on graphs often blur the boundaries between the traditional Machine Learning categories.
This book provides a synthesis and overview of graph representation learning. It begins with a discussion of the goals of graph representation learning as well as key methodological foundations in graph theory and network analysis. Following this, the book introduces and reviews methods for learning node embeddings, including random-walk-based methods and applications to knowledge graphs. It then provides a technical synthesis and introduction to the highly successful graph neural network (GNN) formalism, which has become a dominant and fast-growing paradigm for Deep Learning with graph data. The book concludes with a synthesis of recent advancements in deep generative models for graphs a nascent but quickly growing subset of graph representation learning.
Скачать Graph Representation Learning
Внимание
Уважаемый посетитель, Вы зашли на сайт как незарегистрированный пользователь.
Мы рекомендуем Вам зарегистрироваться либо войти на сайт под своим именем.
Уважаемый посетитель, Вы зашли на сайт как незарегистрированный пользователь.
Мы рекомендуем Вам зарегистрироваться либо войти на сайт под своим именем.
Информация
Посетители, находящиеся в группе Гости, не могут оставлять комментарии к данной публикации.
Посетители, находящиеся в группе Гости, не могут оставлять комментарии к данной публикации.