- Добавил: tatanavip
- Дата: 1-04-2025, 11:33
- Комментариев: 0
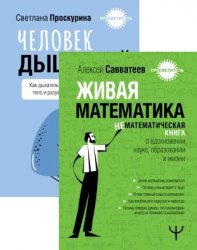
Название: Серия "Просветитель" в 2 книгах
Автор: разные
Издательство: Москва
Год: 2024
Формат: fb2
Размер: 11 Мб
Качество: Хорошее
Язык: Русский
Книги для тех, кто влюблен в науку – понятно, подробно, нетривиально! В книжной серии представлены работы увлеченных популяризаторов, которые доступным языком рассказывают об удивительном мире науки. Книги будут интересны широкой аудитории, которая стремится к знаниям во всем их многообразии!