- Добавил: literator
- Дата: 6-10-2023, 08:55
- Комментариев: 0
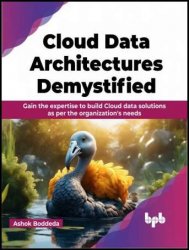
Автор: Ashok Boddeda
Издательство: BPB Publications
Год: 2024
Страниц: 574
Язык: английский
Формат: pdf, epub
Размер: 18.2 MB
Learn using Cloud data technologies for improving data analytics and decision-making capabilities for your organization. Cloud data architectures are a valuable tool for organizations that want to use data to make better decisions. By understanding the different components of Cloud data architectures and the benefits they offer, organizations can select the right architecture for their needs. This book is a holistic guide for using Cloud data technologies to ingest, transform, and analyze data. It covers the entire data lifecycle, from collecting data to transforming it into actionable insights. The readers will get a comprehensive overview of Cloud data technologies and AI/ML algorithms. The readers will learn how to use these technologies and algorithms to improve decision-making, optimize operations, and identify new opportunities. By the end of the book, you will have a comprehensive understanding of loud data architectures and the confidence to implement effective solutions that drive business success. This book is for executives, IT professionals, and data enthusiasts who want to learn more about Cloud data architectures. It does not require any prior experience, but a basic understanding of data concepts and technology landscapes will be helpful.