- Добавил: literator
- Дата: 4-10-2023, 13:36
- Комментариев: 0
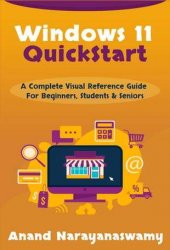
Автор: Anand Narayanaswamy
Издательство: Independently published
Год: 2023
Страниц: 355
Язык: английский
Формат: pdf (true)
Размер: 14.8 MB
Windows 11 QuickStart will be a useful resource for beginners, students, and tech-savvy senior citizens. The book has been authored in such a way that it serves as a perfect resource for anyone who is new to Windows 11 or who wants to learn more about the new operating system. Windows 11 QuickStart provides a detailed and comprehensive overview of the new operating system from Microsoft. Windows 11 QuickStart teaches all the core aspects such as features, design, performance and security of the next-generation Windows 11 operating system in a simple style and language. Windows 11 is a major revamp when compared with Windows 10 and includes brand new features such as a revamped Start menu, Search highlights, Bing Chat, Enhanced Multitasking capabilities, Improved screen capture and much more. The book will serve as a perfect study material for beginners and students including senior citizens who would like to learn the nuances of Windows 11 without any assistance. The aim of the book is to enable students and learners to grasp the concepts easily. The book is written in such a way that you just need to open the book and go through the discussed steps. The book includes several colored screenshots to support the discussed content. Moreover, you will find exclusive content inside boxes that helps you to learn important facts.