Modern Analytics Platforms
- Добавил: literator
- Дата: 13-10-2021, 04:04
- Комментариев: 0
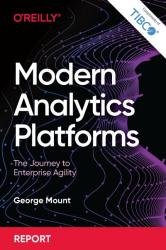
Автор: George Mount
Издательство: O’Reilly Media, Inc.
Год: 2021-10-12
Язык: английский
Формат: epub
Размер: 10.2 MB
As opposed to earlier analytics strategies, IT professionals now seek to collect data as is from any possible source of value. This data can be in a variety of formats, so few predefined rules or relationships are established for ingestion. Depending on the data size, data is processed in batch over discrete time periods, or in streams and events near real time. Because data cleaning is the last step, this process is sometimes referred to as extract-load-transform (ELT), as opposed to the ETL of earlier architectures. For data scientists and other technical professionals, faster access to more and more dynamic data better enables the rapid development of training sets of data for machine learning models. The ELT process allows for the construction of machine learning models, where computers are able to improve performance as more data is passed to them.
As more data is collected and put into production, the importance of a data governance process typically grows, describing who has authority over data and how that data should be used. Similar approaches are necessary to audit how models are put into production and how they work.
In 2011, James Dixon, then chief technology officer of Pentaho, coined the term data lake as the architecture needed to support the next level of analytics maturity.5 Dixon argued that because of the inherently rigid structures of data warehouses, getting value from the increasing volume and variety of data associated with Big Data was difficult. A data lake, “a repository of data stored in its natural/raw format,” was a better approach. In particular, this arrangement wasn’t suited to operate or capitalize on the expanding volume and variety of Big Data.
The data lake is often powered by cloud computing for the benefits of reliability, redundancy, and scalability. Dominant cloud service providers include Amazon Web Services (AWS), Microsoft Azure, and Google Cloud Platform (GCP). Open source technologies like Hadoop and Spark are used to process and store massive datasets using parallel computing and distributed storage. Because this data is often unstructured, it may be stored in graph, document, or other nonrelational databases.
With the increasing volume and velocity of data, and the use of data lakes along with data warehouses to enable data-driven decisions, businesses needed better ways to scale and share business intelligence. One such path was through interactive, immersive exploration and visualization of the data, as pioneered with Spotfire. Other paths were through visual reports and dashboards, as used by not just Spotfire, but by Jaspersoft, Power BI, WebFOCUS, and many others.
If maintaining legacy analytics is like raising a thoroughbred, then developing converged analytics is like cultivating a school of goldfish. That is, the backend provisioning is no longer served by monolithic systems but rather by composable groups of microservices. This arrangement supports elastic and scalable analytics; composability makes it easier to adapt to changes driven in part by a growing volume and variety of data sources.
Скачать Modern Analytics Platforms
Внимание
Уважаемый посетитель, Вы зашли на сайт как незарегистрированный пользователь.
Мы рекомендуем Вам зарегистрироваться либо войти на сайт под своим именем.
Уважаемый посетитель, Вы зашли на сайт как незарегистрированный пользователь.
Мы рекомендуем Вам зарегистрироваться либо войти на сайт под своим именем.
Информация
Посетители, находящиеся в группе Гости, не могут оставлять комментарии к данной публикации.
Посетители, находящиеся в группе Гости, не могут оставлять комментарии к данной публикации.