Mining of Massive Datasets
- Добавил: Igor1977
- Дата: 10-08-2019, 06:35
- Комментариев: 0
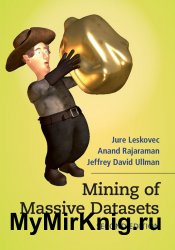
Название: Mining of Massive Datasets
Автор: Leskovec J., Rajaraman A., Ullman J.D.
Издательство: Stanford University
Год: 2019
Формат: pdf
Страниц: 603
Для сайта: LitMy
Размер: 18 mb
Язык: английский
This book evolved from material developed over several years by Anand Rajaraman and Jeff Ullman for a one-quarter course at Stanford. The course CS345A, titled “Web Mining,” was designed as an advanced graduate course, although it has become accessible and interesting to advanced undergraduates. At the highest level of description, this book is about data mining. However, it focuses on data mining of very large amounts of data, that is, data so large it does not fit in main memory. Because of the emphasis on size, many of our examples are about the Web or data derived from the Web. Further, the book takes an algorithmic point of view: data mining is about applying algorithms to data, rather than using data to “train” a machine-learning engine of some sort.
The principal topics covered are:
Distributed file systems and map-reduce as a tool for creating parallel algorithms that succeed on very large amounts of data.
Similarity search, including the key techniques of minhashing and locality-sensitive hashing.
Data-stream processing and specialized algorithms for dealing with data that arrives so fast it must be processed immediately or lost.
The technology of search engines, including Google’s PageRank, link-spam detection, and the hubs-and-authorities approach.
Frequent-itemset mining, including association rules, market-baskets, the A-Priori Algorithm and its improvements.
Algorithms for clustering very large, high-dimensional datasets.
Two key problems for Web applications: managing advertising and recommendation systems.
Algorithms for analyzing and mining the structure of very large graphs, especially social-network graphs.
Techniques for obtaining the important properties of a large dataset by dimensionality reduction, including singular-value decomposition and latent semantic indexing.
Machine-learning algorithms that can be applied to very large data, such as perceptrons, support-vector machines, gradient descent, and decision trees.
Neural nets and deep learning, including the most important special cases: convolutional and recurrent neural networks, and long short-term memory networks.
To appreciate fully the material in this book, we recommend the following prerequisites:
An introduction to database systems, covering SQL and related programming systems.
A sophomore-level course in data structures, algorithms, and discrete math.
A sophomore-level course in software systems, software engineering, and programming languages.
The book contains extensive exercises, with some for almost every section. We indicate harder exercises or parts of exercises with an exclamation point. The hardest exercises have a double exclamation point.
Preface.
Data Mining.
MapReduce and the New Software Stack.
Finding Similar Items.
Mining Data Streams.
Link Analysis.
Frequent Itemsets.
Clustering.
Advertising on the Web.
Recommendation Systems.
Mining Social-Network Graphs.
Dimensionality Reduction.
Large-Scale Machine Learning.
Neural Nets and Deep Learning.
Index.

Внимание
Уважаемый посетитель, Вы зашли на сайт как незарегистрированный пользователь.
Мы рекомендуем Вам зарегистрироваться либо войти на сайт под своим именем.
Уважаемый посетитель, Вы зашли на сайт как незарегистрированный пользователь.
Мы рекомендуем Вам зарегистрироваться либо войти на сайт под своим именем.
Информация
Посетители, находящиеся в группе Гости, не могут оставлять комментарии к данной публикации.
Посетители, находящиеся в группе Гости, не могут оставлять комментарии к данной публикации.