- Добавил: Igor1977
- Дата: 29-09-2021, 11:37
- Комментариев: 0
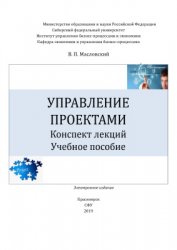
Название: Управление проектами. Конспект лекций
Автор: Масловский В.П.
Издательство: Красноярск: Сибирский федеральный университет
Год: 2019
Формат: pdf
Страниц: 360
Размер: 16 mb
Язык: русский
Изложена теория управления проектами и рассмотрен практический инструментарий проектного менеджмента. Приведён анализ международных стандартов по управлению проектами. Рассмотрены вопросы, охватывающие основные области знаний международных и отечественных стандартов в сфере управления проектами.