Model Order Reduction: Volume 3 Applications
- Добавил: literator
- Дата: 10-06-2021, 13:03
- Комментариев: 0
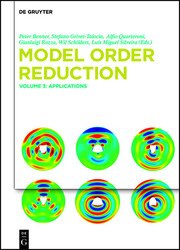
Автор: Peter Benner, Stefano Grivet-Talocia, Alfio Quarteroni
Издательство: De Gruyter
Год: 2021
Страниц: 474
Язык: английский
Формат: pdf (true), epub
Размер: 78.4 MB
An increasing complexity of models used to predict real-world systems leads to the need for algorithms to replace complex models with far simpler ones, while preserving the accuracy of the predictions. This three-volume handbook covers methods as well as applications. This third volume focuses on applications in engineering, biomedical engineering, computational physics and computer science.
The third volume of the Model Order Reduction handbook project offers several remarkable instances of applications of model order reduction (MOR) approaches to the solution of problems arising from the most diverse areas of application. Through these examples, we would like to provide the reader with an overview of the maturity of this emerging field and its readiness to address challenging problems of multifaceted complexity.
We start with several chapter contributions to classical fields of engineering.
The first one, by J. Eason and L. Biegler, is on model reduction in the optimization of a variety of heterogeneous chemical processes. In particular, two case studies are presented on CO2 capture using nonlinear programming and NLP filter models.
The second chapter, by B. Lohmann et al., is on MOR in mechanical engineering. Four applications are discussed, concerning the reduction of a thermo-mechanical machining tool of a car body and driver’s seat, of an elastic crankshaft, and a leaf spring model.
The third chapter, by E. Deckers et al., presents several case studies of MOR for acoustics and vibrations in mechanical applications. Two different viewpoints are developed: the application of MOR from a purely mathematical perspective and a consideration of expected properties of MOR based on physical arguments from the field of mechanics.
Two chapters devoted to microelectronics and electromagnetism, a very classical and successful arena for MOR methods, follow. The first of those, by B. Nouri et al., pursues a twofold goal: to describe the context in which the need for MOR arose in microelectronics, and to present an overview of their applications to address the issues of high-speed interconnects in microelectronics at various levels of the design hierarchy.
The next chapter, by D. Ioan et al., proposes a computer-aided consistent and accurate description of the behavior of electromagnetic devices at various speeds or frequencies, and describes procedures to generate compact electrical circuits featuring an approximately equivalent behavior.
The chapter by M. Yano is on model reduction in computational aerodynamics. The focus is on techniques that are designed to address nonlinearity, limited stability, limited regularity, and a wide range of scales that have been demonstrated successful for multidimensional large-scale aerodynamic flows.
The next two chapters address a somehow less conventional field of applications, that of life sciences. The chapter by B. Karasözen is on MOR in neurosciences, more specifically on the exploitation of models of large-scale neuronal networks to provide an accurate and fast prediction of patterns and their propagation in different areas of the brain.
The following chapter, by N. Dal Santo et al., introduces MOR methods to face some of the most challenging processes of the cardiovascular system. Two specific applications are targeted: the simulation of blood flow past a carotid bifurcation and the computation of activation maps in cardiac electrophysiology.
The last five chapters address somewhat more methodological issues arising in various scientific, engineering, societal, and economics applications.
The chapter by J.-C. Loiseau aims at bypassing some difficulties of classical proper orthogonal decomposition approaches to the solution of fluid dynamics problems by using feature-based manifold modeling in which the low-dimensional attractor and nonlinear dynamics are characterized from experimental dаta: time-resolved sensor data and optional nontime-resolved particle image velocimetry snapshots.
In the chapter by R. Pulch, MOR is used in the framework of uncertainty quantification. Established methods like polynomial chaos, stochastic Galerkin, stochastic collocation, and quadrature sampling are reviewed for dynamical systems consisting of ordinary differential equations or differential algebraic equations. Demonstration of applicability is provided on test examples.
The chapter by X. Cheng et al. addresses MOR methods for networks that describe a wide class of complex systems composed of many interacting subsystems. First, clustering-based approaches are reviewed, with the aim of reducing the network scale. Then, methods based on generalized balanced truncation that reduce interconnection structures of a network and the dynamics of each subsystem are discussed.
The chapter by D. Hartmann et al. presents use cases where MOR is a key enabler for the realization of digital services and the reduction of simulation times and outlines the potential of MOR in the context of realizing the digital twin vision.
The last chapter, by B. Haasdonk, addresses the issue of software. In the first part, as neither full simulation models nor MOR algorithms are to be reprogrammed, but ideally are reused from existing implementations, the interplay of such packages is discussed. Then an overview of the most popular MOR software libraries is provided.
Скачать Model Order Reduction: Volume 3 Applications
Внимание
Уважаемый посетитель, Вы зашли на сайт как незарегистрированный пользователь.
Мы рекомендуем Вам зарегистрироваться либо войти на сайт под своим именем.
Уважаемый посетитель, Вы зашли на сайт как незарегистрированный пользователь.
Мы рекомендуем Вам зарегистрироваться либо войти на сайт под своим именем.
Информация
Посетители, находящиеся в группе Гости, не могут оставлять комментарии к данной публикации.
Посетители, находящиеся в группе Гости, не могут оставлять комментарии к данной публикации.